Clinical trials of therapeutics for Alzheimer's Disease (AD) have been unable to obtain significant progress, largely because its disease mechanism and origins remain poorly understood. While it is likely that the misfolding of proteins aggregates as culprits causing neurodegeneration (including AD) (Soto and Pritzkow, 2018), and the synergistic effect between beta-amyloid plaque and tau protein neurofibrillary tangle formation (Bushe and Hyman, 2020) has associations with AD, little connection with medication and therapy have been successfully established. However, with the new machine learning framework of Drug Repurposing in Alzheimer’s Disease (DRIAD), the genes and proteins related to the disease mechanism may be located, whilst potential drug candidates to be repurposed for AD can be identified.
Differential expression of genes via drug treatment
Gene expression is the process by which the instructions in our DNA are converted into a functional product, possibly a protein. It is a form of demonstrating the impact of external factors on cells and genes.
In the framework of DRIAD, two types of input are required: the drug-associated gene list (DGL), and the level of AD via mRNA expression. We shall get to that later.
In order to generate DGLs in this experiment, neural progenitor cells (in simpler terms, the stem cells in the brain that generate different neuron cells) undergo two stages of differentiation: the first stage by a standard differentiation medium to obtain a mixed cell population, and the second by a certain drug from the panel of candidate drugs to be potentially used for repurposing. Through data analysis and the comparison of gene expression levels between stages and standard values, DGLs for the particular drug are obtained, meaning that the genes in the DGL are affected by the molecular mechanisms of the drug to show a significant difference in gene expression level. This means that each drug has its own DGLs. At the same time, we are unable to determine whether the impact of the drug on the genes involved are ultimately beneficial or harmful to the therapeutics of AD; we can only interpret the presence of the impact and its extent by the perturbation caused on the gene expression profile of the cells.
DGL and AD stage predictor
The gene expression profile (mRNA, the product of DNA transcription) from the brain of an AD patient gives information to the stage of AD the brain is in. The stage of AD is defined by the Braak stage, where scores are given to different DNA expression profiles to quantify the stage of AD. In this machine learning framework, the stage of AD is classified into early (A), middle (B) and late (C).
In the machine learning framework, a particular DGL is subsampled and selected. It then undergoes a statistical process known as cross-validation to be trained as a predictor for the stage of AD. In cross-validation, the DGL is first exposed to a set of data of known stage of AD (via the gene expression level aforementioned) where it is trained to learn the scores of the Braak stage for the data it receives. It is then exposed to a new set of data where the list needs to determine the stage of AD of the specimen through its mRNA expression. In particular, data analysis was focused on distinguishing the two extreme stages, A and C, since the more distinct differences in mRNA expression level implies a higher ability of random lists to distinguish between the two stages and a DGL would have to perform considerably better in order to be considered statistically significant in performance.
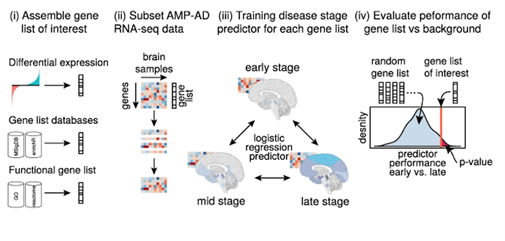
Fig. 1: Illustration of the working principle of DRIAD
The DGL’s accuracy of performance in identifying the AD stage of the specimen unknown to the list is recorded and compared against various other randomly selected DGLs of the same length. When a DGL has a relatively high accuracy in identifying the AD stage compared to lists of equal length (it outperforms random lists), it suggests that the genes and gene lists involved may have a high degree of association to the occurrence of AD. Figure 2 on the right shows the performance of a few gene lists compared over the curve of the performance of random gene lists of the same length. Different colours represent databases taken from different parts of the brain.

Fig. 2
Through the repetition of the process with various DGLs of various lengths generated from various drug candidates, the drugs whose DGLs develop predictors demonstrating a consistently accurate performance in identifying the stage of AD may suggest that the drug has a relatively strong association with AD mechanisms, and they are ranked accordingly (fig. 3).
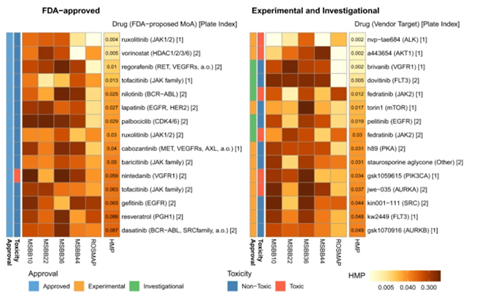
Fig. 3: Drugs ranked in order of overall performance of their DGLs in predicting AD stage. HMP refers to the “Harmonic mean P-value”, the lower the HMP, the lower the proportion of gene lists that outperformed the DGLs. The bracket indicates the primary target of the drug, but is not conclusive due to polypharmacology of drugs (explained below).
Primary results and further investigation
By analysing the results of predictors trained from DGLs of each drug, the drugs and chemical compounds can be ranked, and higher-ranking drugs could potentially be repurposed for AD therapeutics.
Many of the higher-ranked drugs are cytotoxic (e.g. nvp-tae684, an inhibitor of anaplastic lymphoma kinase, an enzyme essential for cellular communication), meaning they are unlikely to be useful for further development into a mature AD therapeutic. This, however, may indicate in the opposite direction that the chemical action of these drugs on neuronal cells may be similar to the mechanism of cell death in the brain that leads to AD, providing a direction for further investigation on AD mechanisms.
As for drugs that are non-toxic (e.g. ruxolitinib, originally used to treat a rare type of blood cancer where the bone marrow is replaced by fibrous tissues), not only can they be studied further for direct repurposing for AD treatment, but also, along with toxic drugs, be put under the study of the common targets of these drugs (i.e. chemicals and compounds that the drugs target on modifying, transforming or removing) by investigating the bond affinity of drugs with these targets. These targets can include proteins, enzymes, genes, hormones, etc. Since higher-ranked drugs are deduced to be closely related to the AD mechanism, the common targets of these higher-ranked drugs may play a potential role in causing AD.
These targets includes JAK kinases (affecting JAK-STAT interferon signaling in the brain), RPS6KA2 (associated with Parkinson’s Disease (PD) – this may imply certain similarities between PD and AD mechanisms), and NIMA-related kinases (disrupting microtubules – microscopic hollow tubes made of the proteins forming the cell skeleton, and causing formation of neurofibrillary tangles that are known to cause AD). With some of these targets previously confirmed to be associated with AD, we can both confirm the validity of the DRIAD framework in relation to the drivers of AD, and be able to stimulate the molecular mechanism of these targets outside of the human body using the drugs, which may possibly reveal more of the true mechanism, or even the origins, of AD.
Evaluating its implications, advantages and limitations
In the past, various studies have been conducted on AD. Whilst returning promising results on the mechanism itself, no comprehensive explanation has been made on the origins of these processes, for instance, the misfolding of protein aggregates, or formation of beta-amyloid plaques in the blood-brain barrier that hinders neuronal communications and impede synaptic connections. (Bushe and Hyman, 2020) As a result, minimal association could be made between these phenomena observed and drugs and therapeutics, let alone early diagnosis. In this DRIAD framework, we reverse the process of investigation: instead of using observed changes to the brain to explain the mechanisms and find medical solutions, we use the trial of medical solutions to stimulate what changes occur in the brain and attempt to explain the underlying pathogenesis – the disease mechanisms which are still poorly understood to this day, and, more importantly, medical solutions are simultaneously derived and the association between drugs and the disease is effectively established; this proves to be a new direction in the study of AD and improvement of its treatments.
As mentioned above, the effect of drugs on genes are two-sided, since the ultimate result of the drug’s perturbation on gene expression is unexplored. That means both disease-enhancing and disease-reducing drugs are included in the list. However, regardless of whether they could be used directly for repurposing, they all help to unravel the pathogenesis of AD. The advantage of this machine learning framework is that it returns two levels of experimental outcome – whether a drug can be repurposed, and the association between the chemical action of drugs on genes and the modification of genes in an AD case.
By using chemical compounds as the starting point for investigation, more diverse mechanisms potentially leading to the incurrence of AD previously unexplored can be revealed. These biological processes, like autophagy, the process of cleaning out damaged cells and removing neurotoxic aggregates, have not been considered as highly associated with the occurrence of AD in previous studies due to a lack of evidence. They can be identified under DRIAD as being associated with AD due to the polypharmacology of drugs – the nature of drugs targeting an unknown number of multiple targets. As a result, future studies may investigate the possibility of controlling such biological processes as a method to cure AD, for example increasing autophagy to clear neurotoxic substances using the top-ranked drugs.
Finally, by drug-driven investigation, the well-understood mechanisms of drugs establish a correlation of AD with different diseases. For example, it is likely that there is an inverse relationship of the occurrence of AD with cancer, supported by the evidence that JAK-STAT interferon communications, the disruption of which is known to cause cancer, is inhibited by many of the top-ranked drugs. The pathology of AD in relation to the neuronal cause of cancer may hence open up a potential direction for investigation of its pathogenesis. With all the benefits above, it is almost certain that the use of the DRIAD framework will provide more substantial evidence on the understanding of the disease itself and the therapeutics that can solve this issue, which has been almost exasperating to the medical world.
Article written based on, and graphs obtained from: Rodriguez, S., Hug, C., Todorov, P., Moret, N., Boswell, S. A., Evans, K., Zhou, G., Johnson, N. T., Hyman, B T., Sorger, P. K., Albers, M. W., & Sokolov, A. (2021). Machine learning identifies candidates for drug repurposing in Alzheimer’s Disease. Nature Communications, 12:1033. https://doi.org/10.1038/s41467-021-21330-0.
Citations:
1. Bushe, M. A., & Hyman, B. T. (2020). Synergy between amyloid-𝛃 and tau in Alzheimer’s Disease. Nature neuroscience. https://doi.org/10.1038/s41593-020-0687-6.
2. Soto, C., & Pritzkow, S. (2018, October). Protein misfolding, aggregation and conformational strains in neurodegenerative diseases. Nature neuroscience, Vol. 21, 1332-1340. https://doi.org/10.1038/s41593-018-0235-9.
Comments